Embracing the Future: How Machine Learning and AI Transform Intrusion Detection
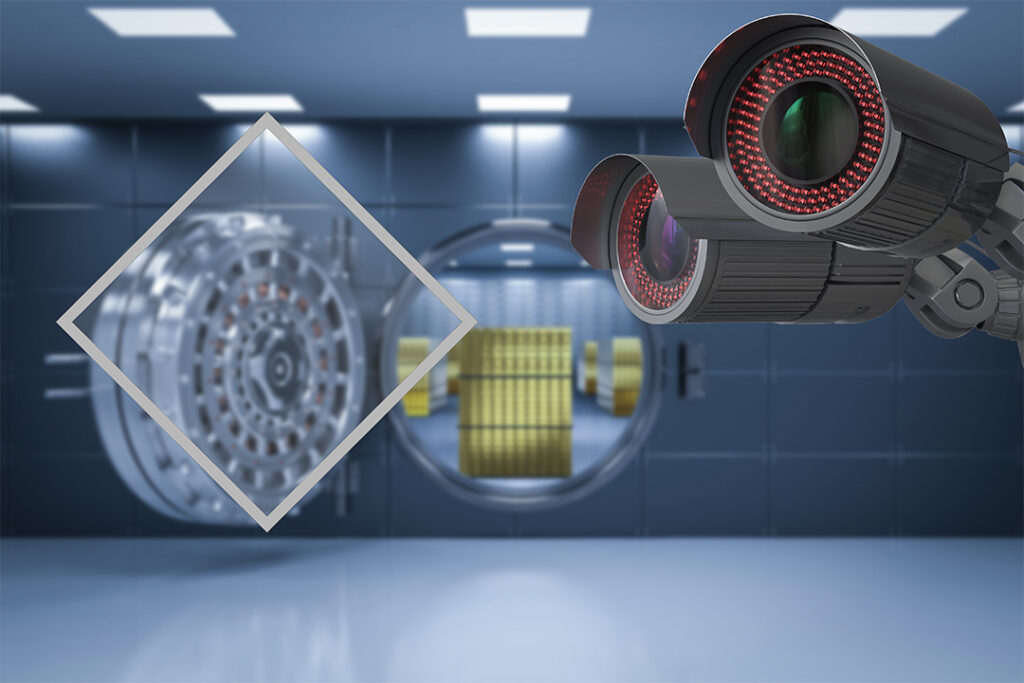
In the perpetual game of cat and mouse between cyber attackers and defenders, organizations are increasingly turning to cutting-edge technologies like Machine Learning (ML) and Artificial Intelligence (AI) to bolster their Intrusion Detection Systems (IDS). This blog explores the transformative impact of ML and AI on intrusion detection, unraveling the complexities, and unveiling the potential for a more proactive and adaptive cybersecurity defense.
The Evolution of Intrusion Detection
Traditional Intrusion Detection Systems rely on predefined signatures and rules to identify known patterns of attacks. While effective against known threats, they often struggle when faced with the ever-growing sophistication of modern cyber threats. This has paved the way for the integration of ML and AI into intrusion detection strategies.
Harnessing Machine Learning
Anomaly Detection
ML algorithms excel in anomaly detection, a technique that involves learning normal behavior within a network and identifying deviations from this baseline. Abnormal patterns, which may signify potential security threats, can be detected in real-time, providing a proactive defense against novel and previously unseen attacks.
Behavioral Analysis
ML models can analyze user and system behavior to create profiles of typical activities. Deviations from these established behavioral patterns can trigger alerts, helping detect insider threats or unauthorized access.
Dynamic Threat Modeling
ML enables dynamic threat modeling, where the system continuously learns and adapts to the evolving threat landscape. This self-learning capability ensures that the intrusion detection system remains effective against emerging and polymorphic threats.
The Role of Artificial Intelligence
Advanced Pattern Recognition
AI, a broader field encompassing ML, excels in advanced pattern recognition. It can identify subtle, complex patterns in network traffic and user behavior that might evade traditional detection methods.
Decision-Making Capabilities
AI-driven intrusion detection systems can make real-time decisions based on the analysis of vast datasets. This enables rapid responses to potential threats, reducing the window of vulnerability and mitigating the impact of cyber incidents.
Adaptive Defense Mechanisms
AI empowers intrusion detection systems with adaptive defense mechanisms. The system can autonomously adjust its response strategies based on the severity and context of detected threats, enhancing its ability to counteract sophisticated attack techniques.
Benefits of ML and AI in Intrusion Detection
Reduced False Positives
ML and AI algorithms significantly reduce false positives with their ability to understand context and behavior. This ensures that security teams can focus their efforts on genuine threats rather than sifting through a multitude of false alerts.
Early Threat Detection
ML and AI enable early detection of threats, especially those with subtle or evolving patterns. This proactive approach allows organizations to intervene before an attack can escalate, minimizing potential damage.
Continuous Learning
The self-learning nature of ML and AI models means that intrusion detection systems can continuously adapt to new attack vectors. This adaptability is crucial in an environment where cyber threats are constantly evolving.
Scalability
ML and AI-driven intrusion detection systems are scalable and capable of handling large volumes of data. This scalability is essential for organizations with dynamic and growing network infrastructures.
Challenges and Considerations
Data Quality and Quantity
ML and AI models require large, high-quality datasets for effective training. Ensuring the availability of such data can be a challenge for some organizations.
Explainability
Some ML and AI algorithms’ ‘black-box’ nature poses challenges in explaining the rationale behind their decisions. Explainability is crucial for building trust in the system.
Adversarial Attacks
Cyber adversaries increasingly employ sophisticated techniques to manipulate ML and AI models. Intrusion detection systems must be robust enough to withstand adversarial attacks.
Conclusion
The incorporation of Machine Learning and Artificial Intelligence into Intrusion Detection Systems marks a paradigm shift in cybersecurity. These technologies offer a proactive and adaptive defense mechanism against the dynamic landscape of cyber threats. As organizations continue to invest in bolstering their cybersecurity posture, the symbiotic relationship between human expertise and AI-driven intrusion detection promises a formidable defense against even the most sophisticated adversaries. The journey toward a more secure digital future is undeniably intertwined with the relentless pursuit of innovation in intrusion detection technologies.
To ensure your organization is as secure as possible, contact the professionals at WSS Integrated Technologies today!
- The Role of Continuous Monitoring and Analysis in Intrusion Detection - September 6, 2024
- Understanding Crucial Terms and Technologies in Fire Alarm Systems - August 30, 2024
- The Anatomy of a Fire Alarm System: Essential Components for Complete Protection - August 23, 2024